Using topological text analysis for COVID-19 Open Research Challenge
My take on COVID-19 Kaggle challenge analysis of scientific white papers. This research is a first step to help specialist in virusology, pharmacy and microbiology to find answers to the problem
On 12 March the White House and a coalition of leading research groups prepared the COVID-19 Open Research Dataset in response to the coronavirus pandemic. The dataset consists of over 44,000 scholarly articles, including over 29,000 with full text, about COVID-19, SARS-CoV-2, and related coronaviruses. This freely available dataset is provided to the global research community to apply recent advances in natural language processing and other AI techniques to generate new insights in support of the ongoing fight against this infectious disease. Kaggle page for this challenge can be found here: https://www.kaggle.com/allen-institute-for-ai/CORD-19-research-challenge
Calling all subject matter experts
If you are a specialist in virusology, pharmacy or microbiology, we would love to give you FREE access to the below analysis on DataRefiner platform to help speed up scientific research and tackle the virus. Please contact us on ed@datarefiner.com.

The challenge
The challenge have specified a number of questions for the community:
What is known about transmission, incubation, and environmental stability?
What do we know about natural history, transmission, and diagnostics for the virus? What have we learned about infection prevention and control?
What do we know about COVID-19 risk factors?
What have we learned from epidemiological studies?
What do we know about virus genetics, origin, and evolution?
What do we know about the virus origin and management measures at the human-animal interface?
What do we know about non-pharmaceutical interventions?
What is known about equity and barriers to compliance for non-pharmaceutical interventions?
What do we know about vaccines and therapeutics?
What has been published concerning research and development and evaluation efforts of vaccines and therapeutics?
What do we know about diagnostics and surveillance?
What has been published concerning systematic, holistic approach to diagnostics (from the public health surveillance perspective to being able to predict clinical outcomes)?
Data Preprocessing
We used both abstract and full text of the documents for this analysis. Often the documents in this dataset have only abstract or just full text. Merging them together helped to present the full picture.

Abstracts in scientific papers are normally quite complex and one way to analyse them is to split them into sentences. In this case each sentence is a small piece of information, which should be enough for automatic analysis.
This is an example of one of the abstracts:
‘The basic reproduction number of an infectious agent is the average number of infections one case can generate over the course of the infectious period, in a naïve, uninfected population. It is well-known that the estimation of this number may vary due to several methodological issues, including different assumptions and choice of parameters, utilized models, used datasets and estimation period. With the spreading of the novel coronavirus (2019-nCoV) infection, the reproduction number has been found to vary, reflecting the dynamics of transmission of the coronavirus outbreak as well as the case reporting rate. Due to significant variations in the control strategies, which have been changing over time, and thanks to the introduction of detection technologies that have been rapidly improved, enabling to shorten the time from infection/symptoms onset to diagnosis, leading to faster confirmation of the new coronavirus cases, our previous estimations on the transmission risk of the 2019-nCoV need to be revised.’
For our analysis we split it into sentences:
- The basic reproduction number of an infectious agent is the average number of infections one case can generate over the course of the infectious period, in a naïve, uninfected population
- It is well-known that the estimation of this number may vary due to several methodological issues, including different assumptions and choice of parameters, utilized models, used datasets and estimation period
- With the spreading of the novel coronavirus (2019-nCoV) infection, the reproduction number has been found to vary, reflecting the dynamics of transmission of the coronavirus outbreak as well as the case reporting rate
- Due to significant variations in the control strategies, which have been changing over time, and thanks to the introduction of detection technologies that have been rapidly improved, enabling to shorten the time from infection/symptoms onset to diagnosis, leading to faster confirmation of the new coronavirus cases, our previous estimations on the transmission risk of the 2019-nCoV need to be revised
As you can see, each sentence is actually enough to express a piece of information needed for researchers to at least partially answer a question. In total we’ve got 291281 sentences — that is enough for analysis.
Analysis of sentences
All sentences were analysed and presented as a topological map below, each point on the map is one or more sentences, similar sentences are placed close to each other. Clusters are formed automatically and represent different themes in the text.
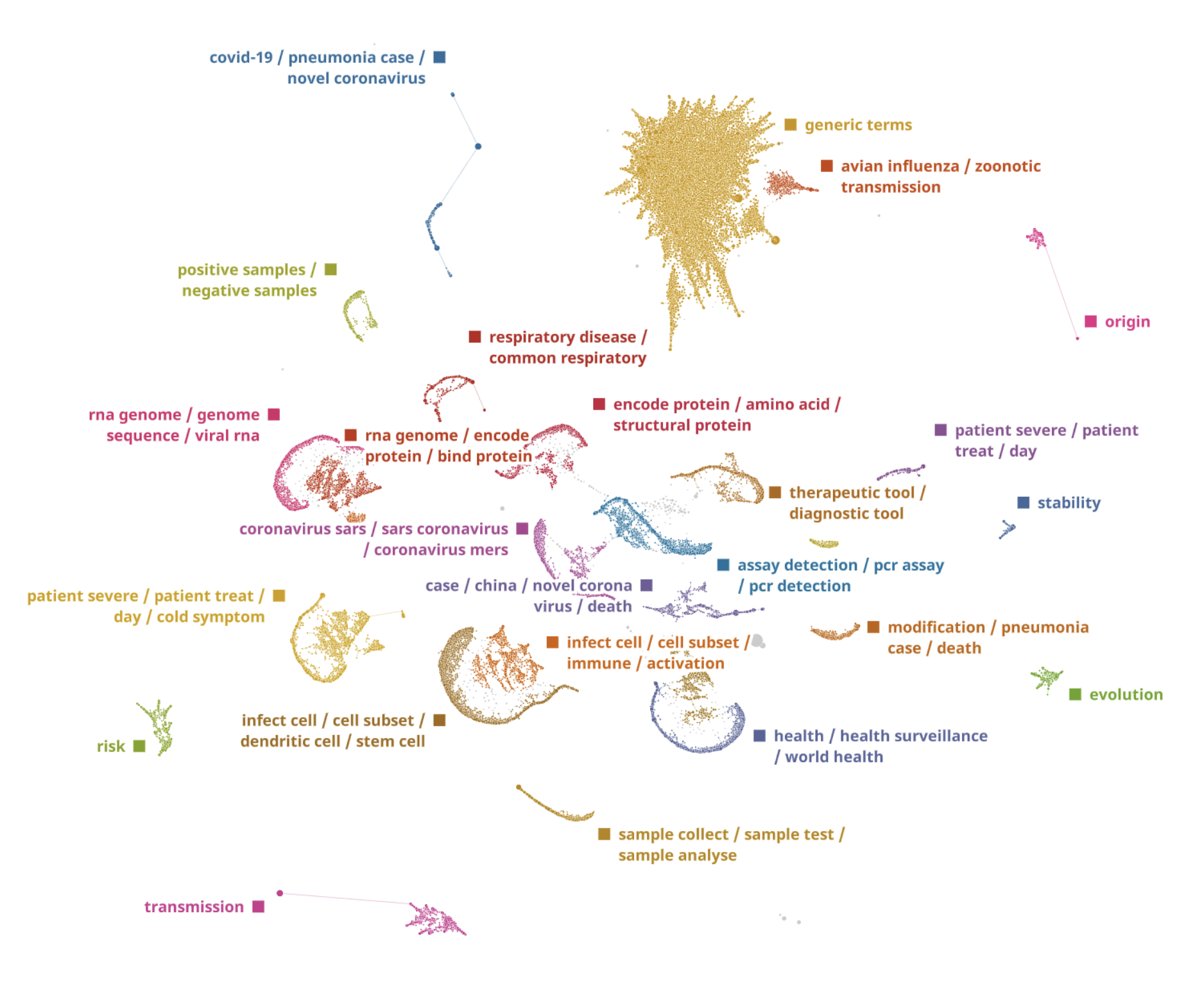
As we trained the model, in addition to automatic clusterisation, we configured it to extract sentences with keywords like “origin”, “stability”, “evolution”, “risk” and “transmission”. We separated these clusters on the map manually using a semi-supervised approach.
Let’s have a look at the “origin” cluster and what knowledge we can extract from it.
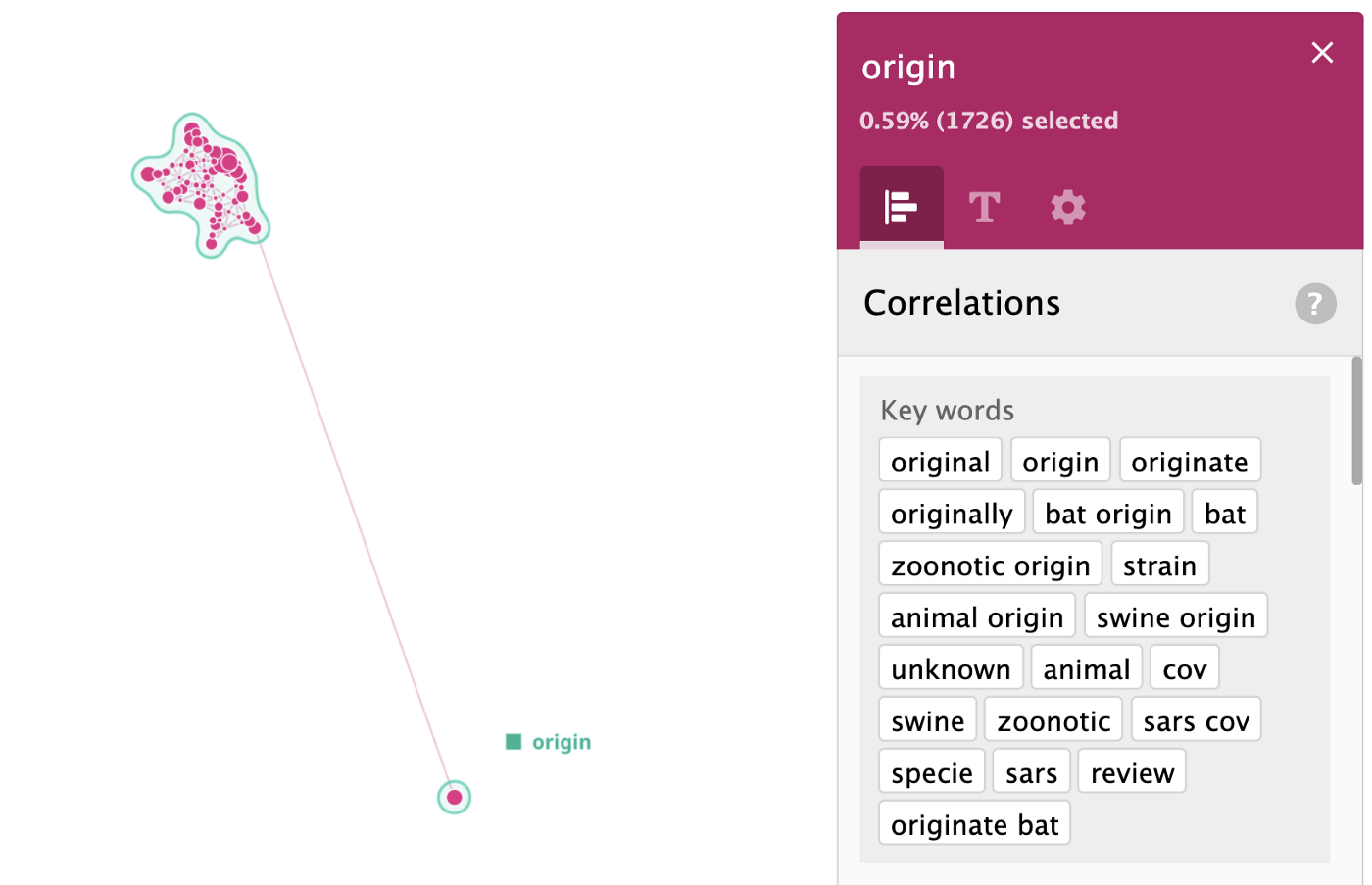
These top keywords were automatically extracted from the sentences, we show top 20 here, the rest will be used to find top summary sentences that describe the cluster the best way.
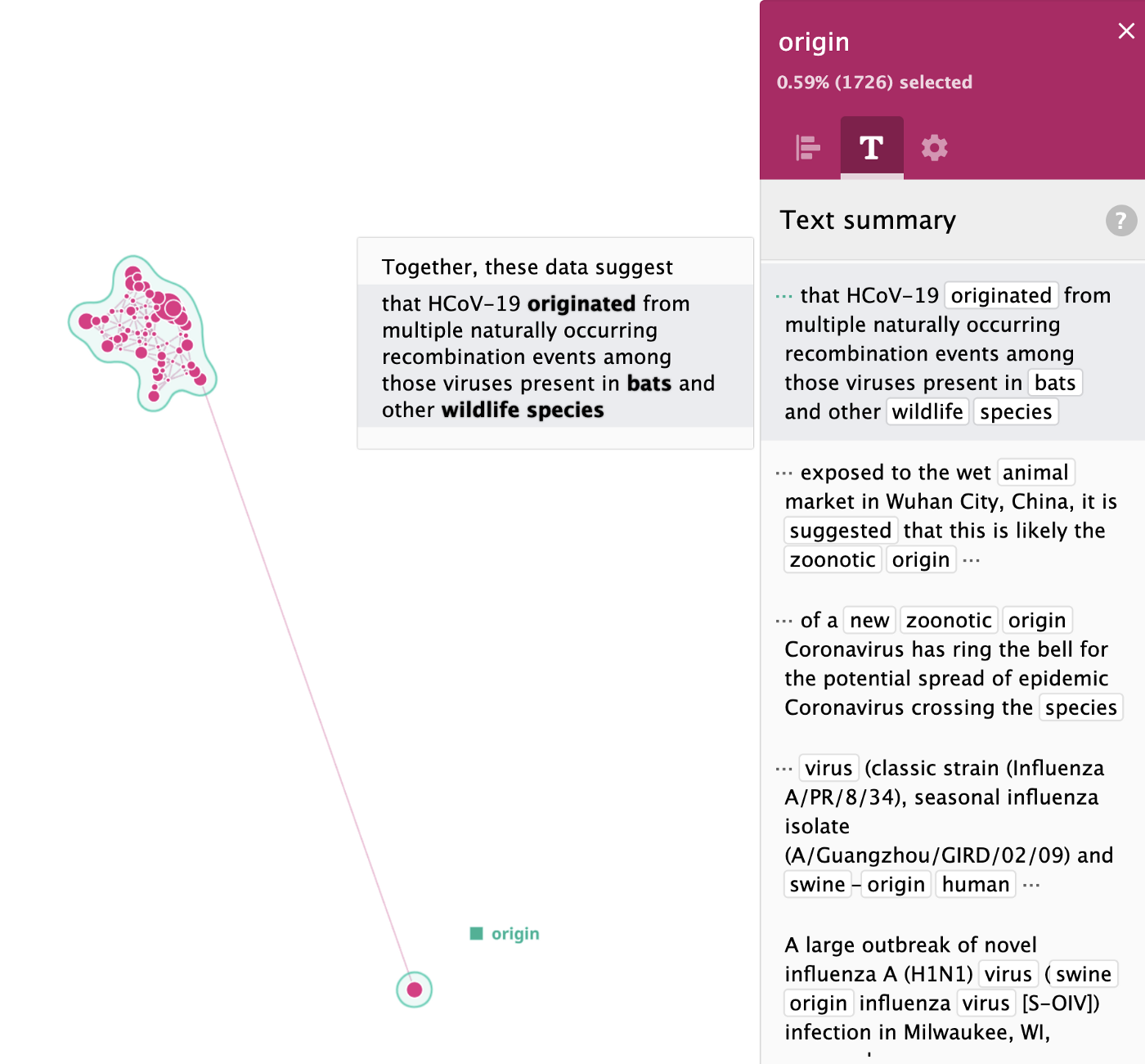
Top sentences describing the cluster ‘origin’ have been identified automatically too and are quite accurate.
Let’s take a look at another cluster, — this time the one, which was formed completely automatically, — “coronavirus sars / sars coronavirus / coronavirus mers”.
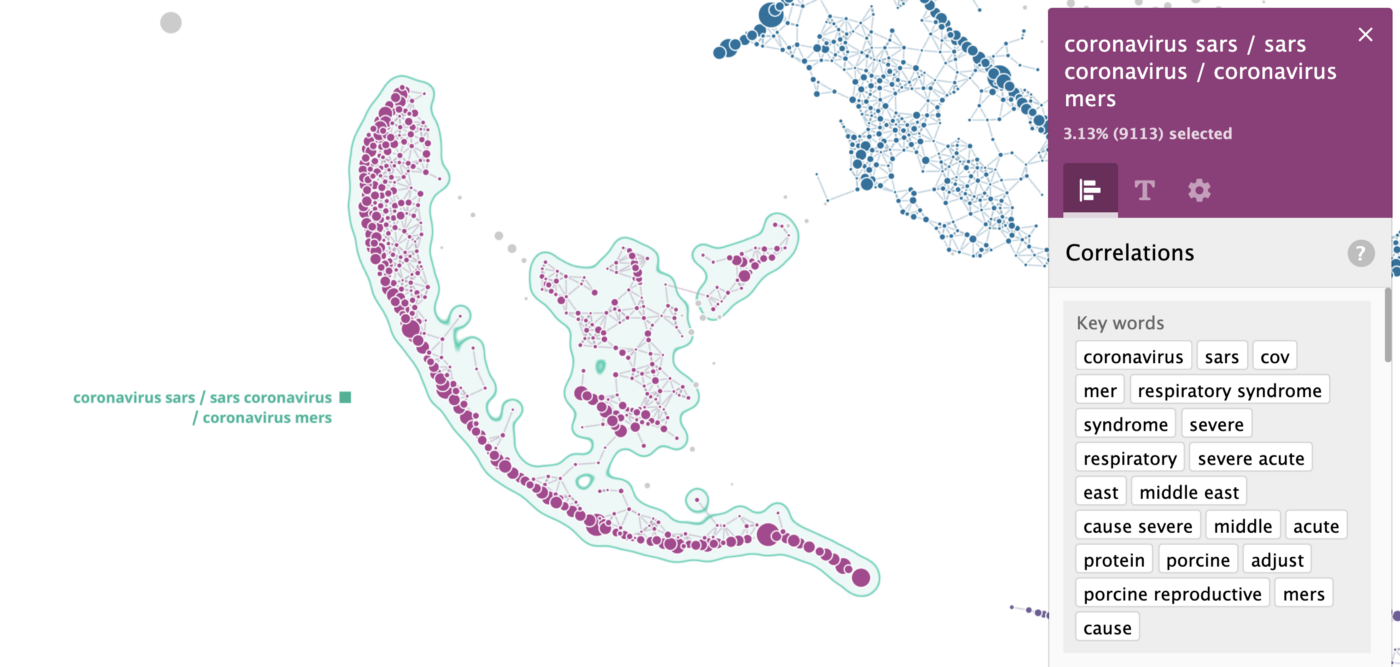
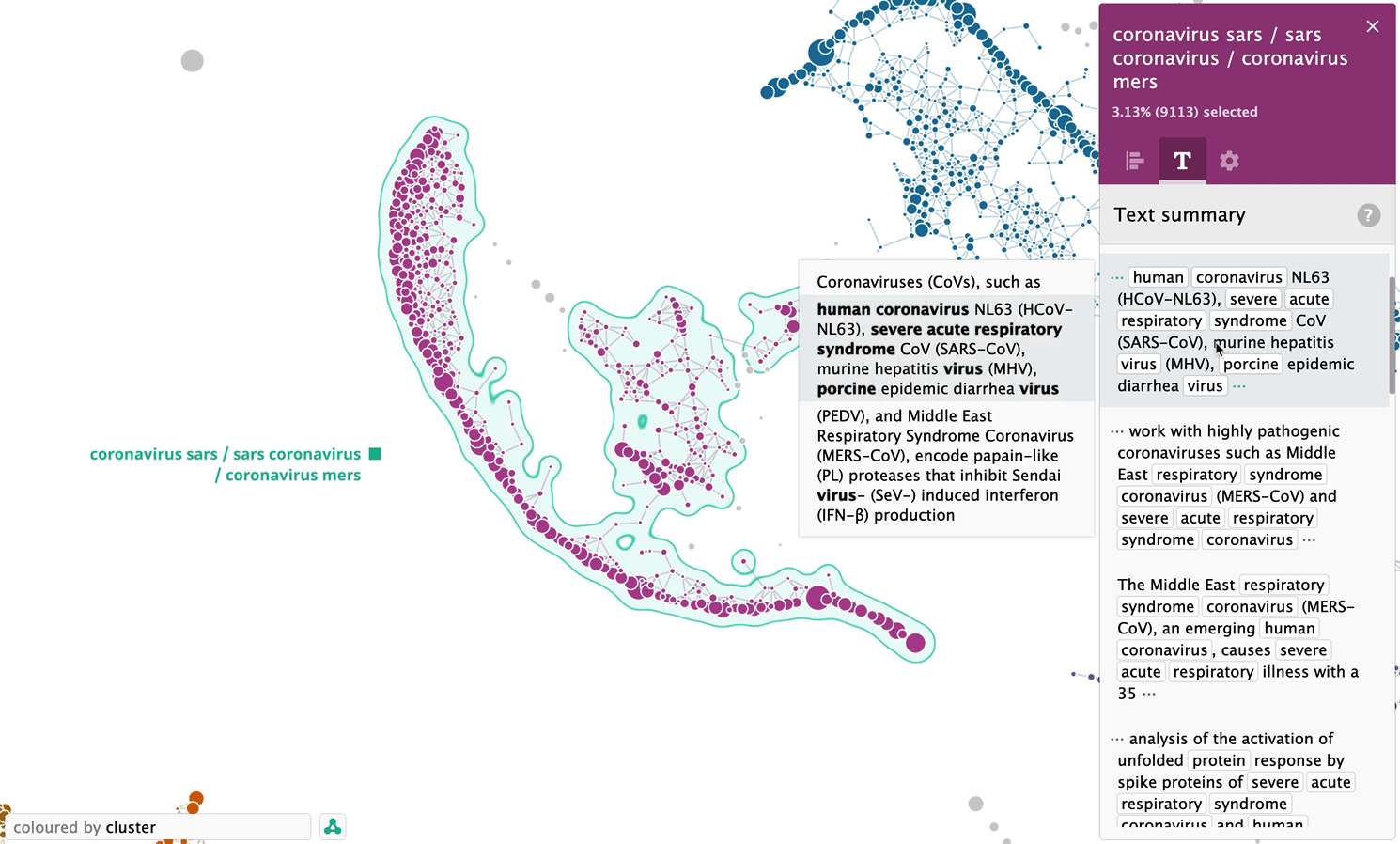
Analysis of sentences related to “transmission” keyword as a separate map
In addition to analysis on a whole set of sentences, the system is able to process sentences related to a particular keyword, for example “transmission”. Below is a map of 8198 sentences related to the keyword “transmission”:
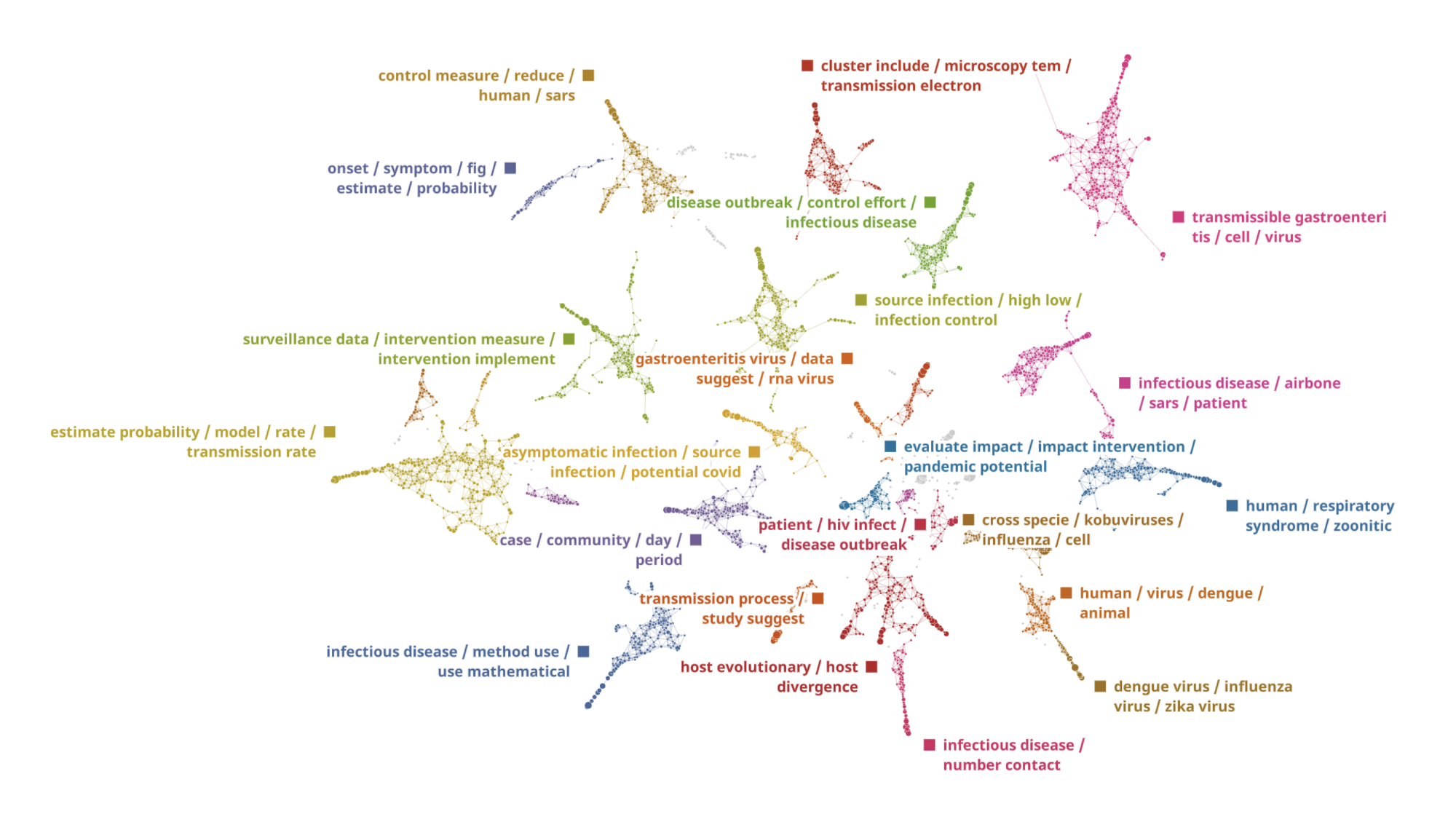
The dataset consists not only of coronavirus white papers but a large number of papers about other recent viruses such as “SARS” (Severe acute respiratory syndrome-related coronavirus), “MARS” (Middle East respiratory syndrome-related coronavirus), “TGEV” Transmissible gastroenteritis virus and some other. We have reviewed different clusters separately to understand all the key nuggets of information about transmission of this and similar diseases.
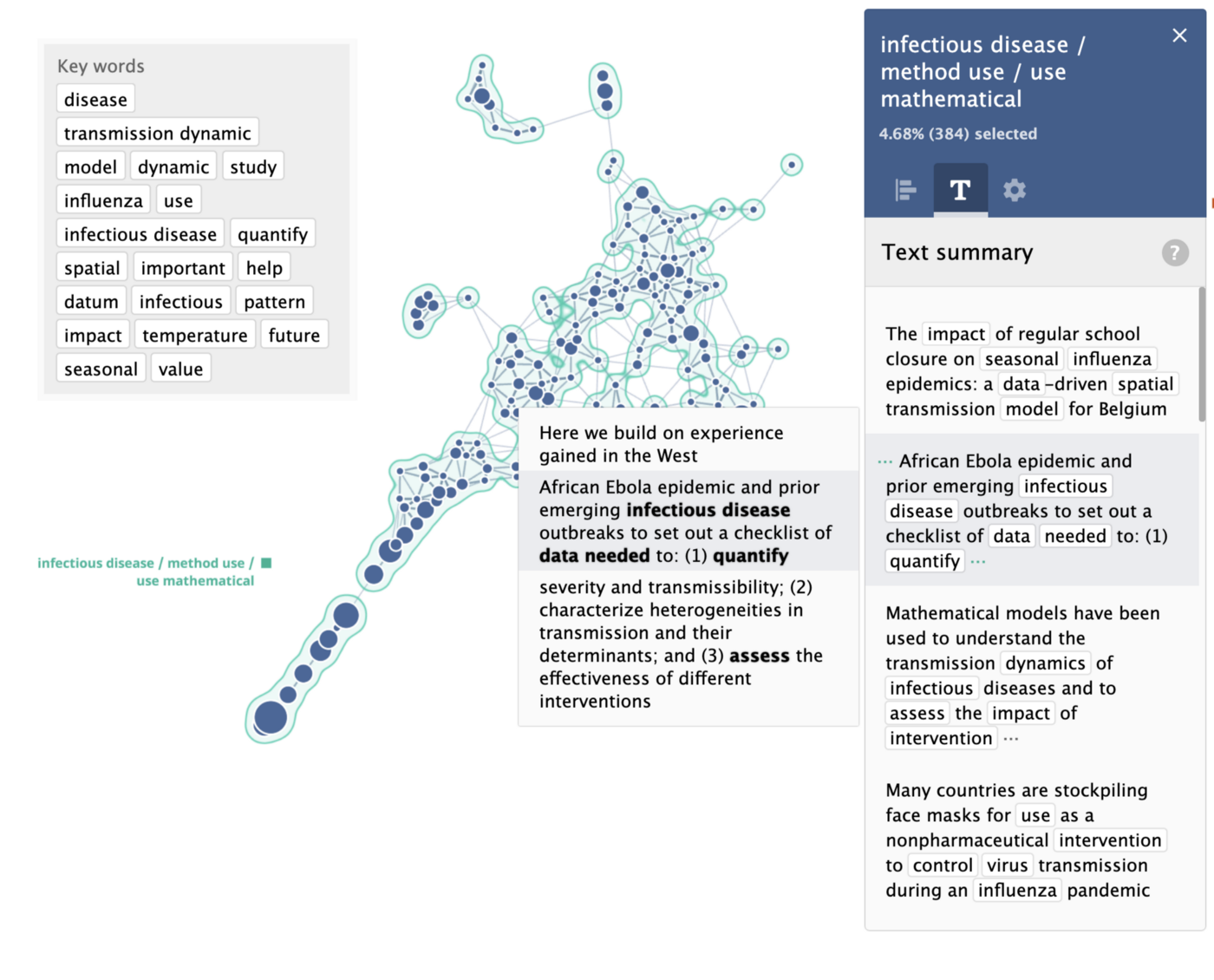
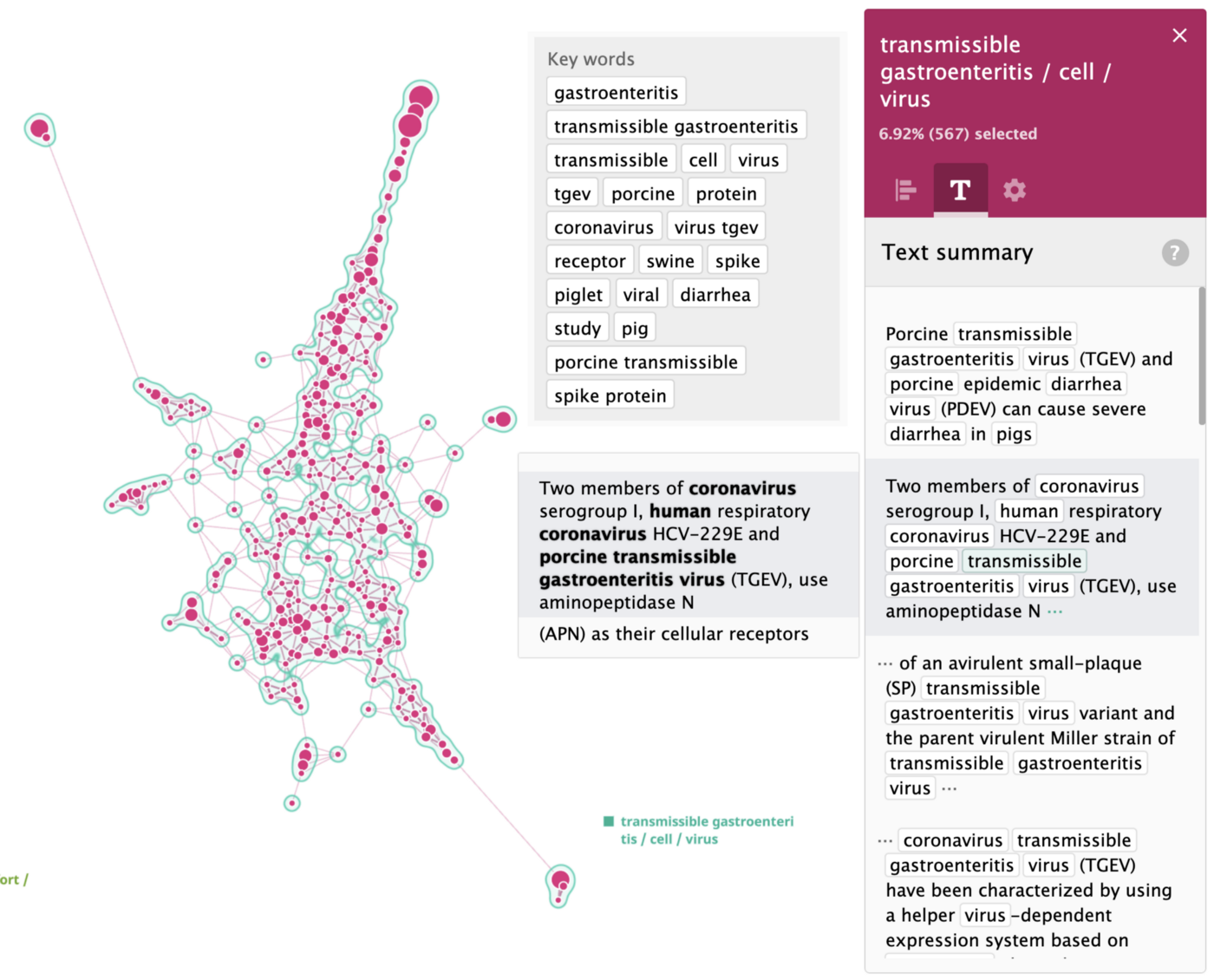
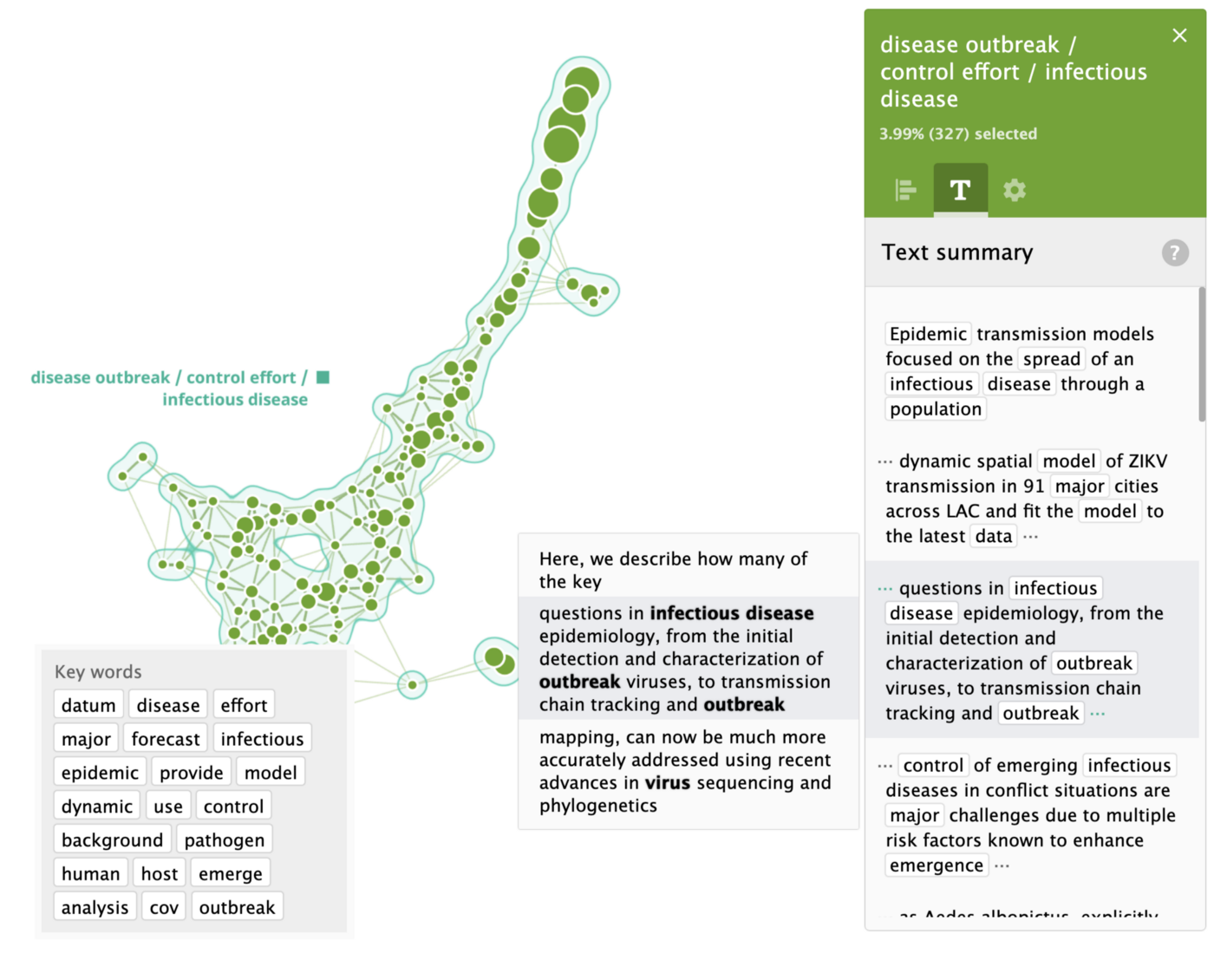
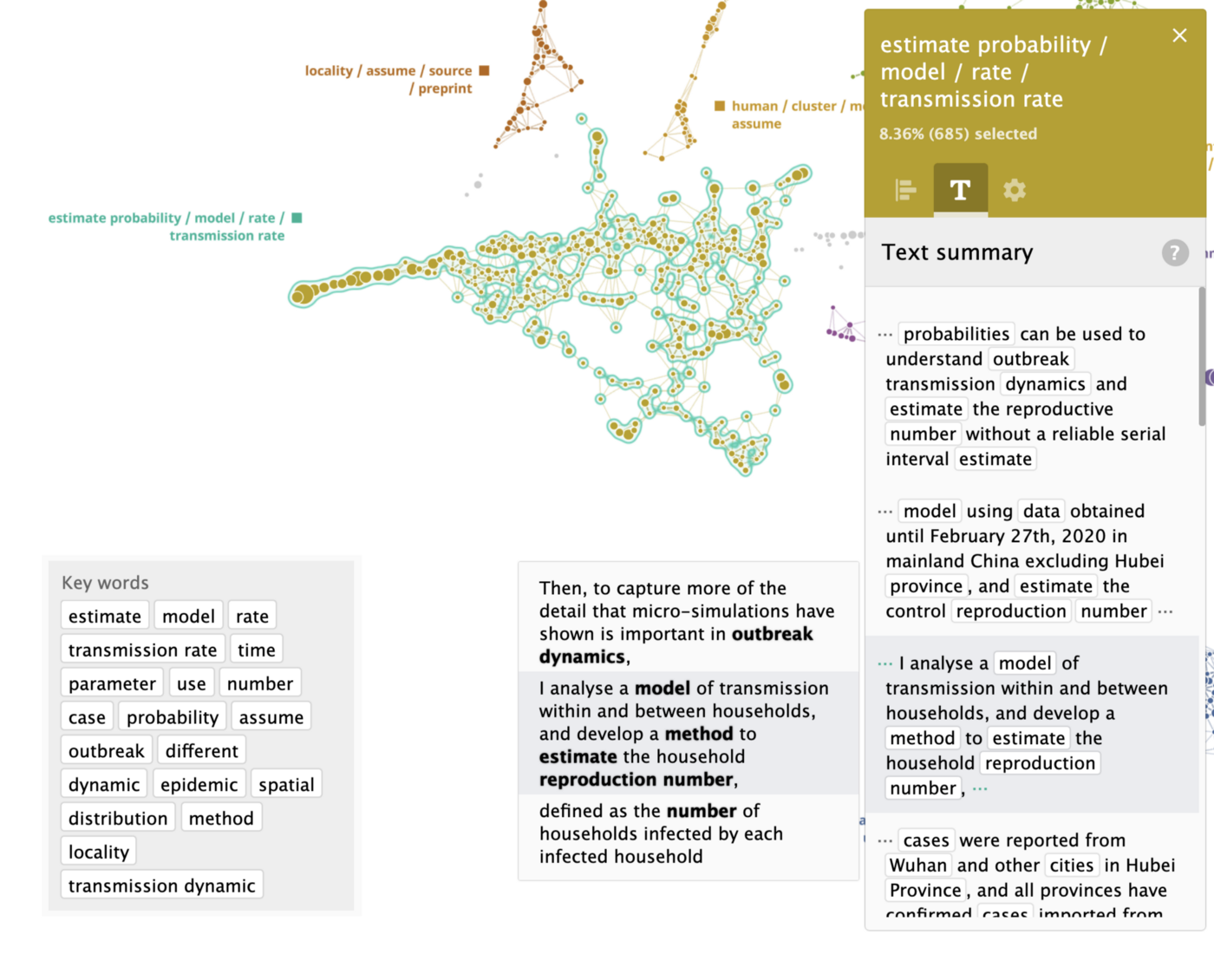
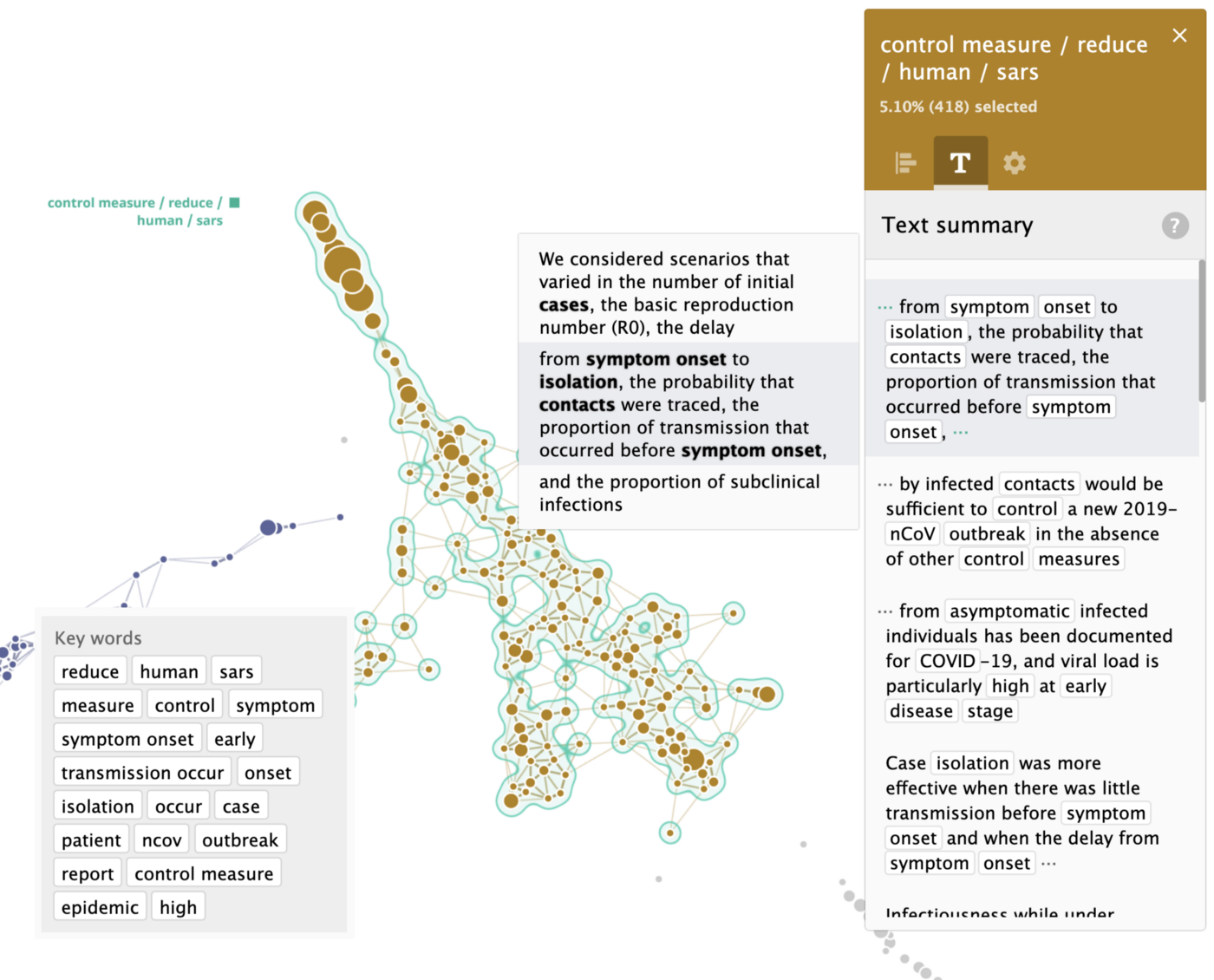
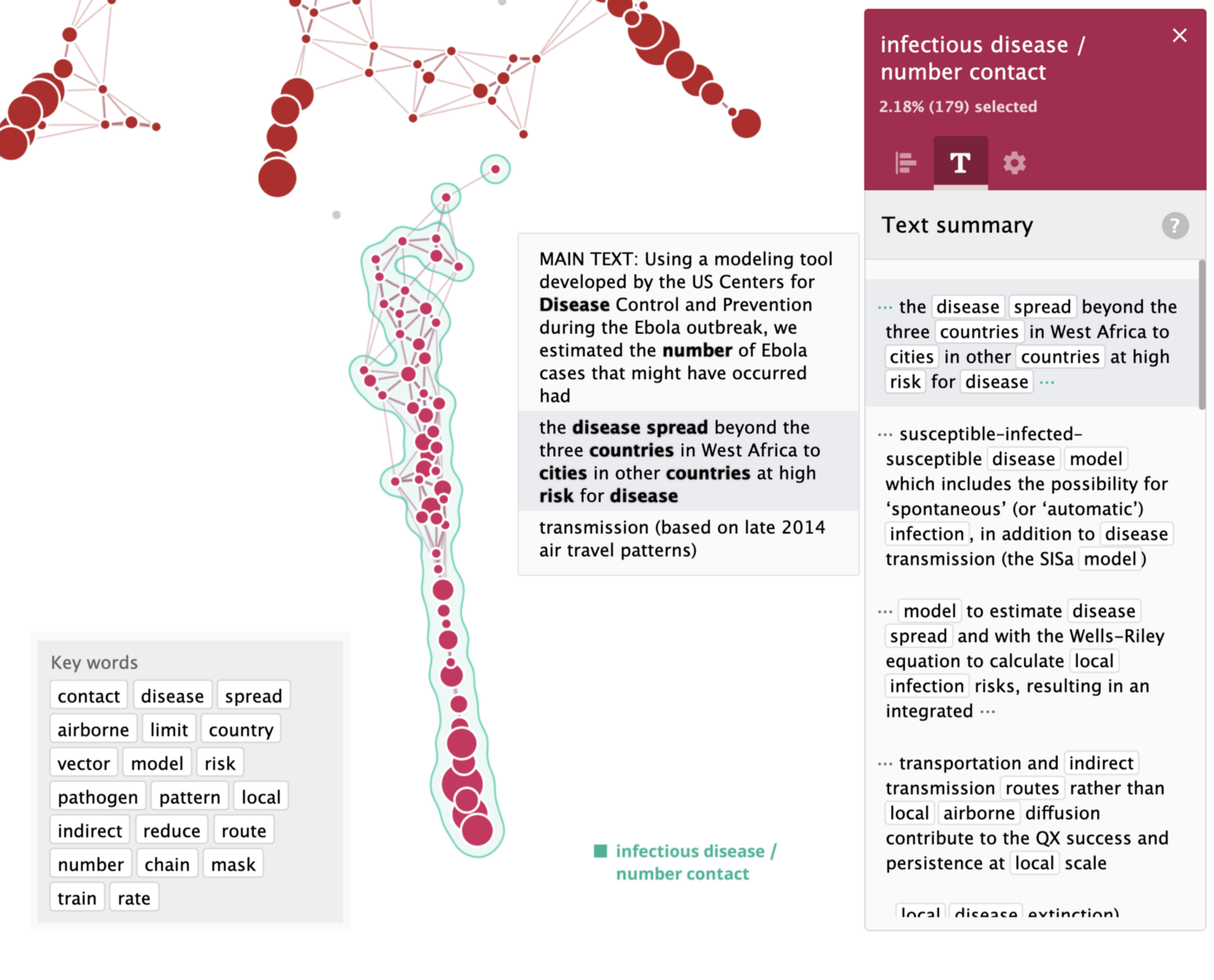
The system automatically identified the clusters of key words and segmented the dataset among them. The closer the clusters are, the more similar terms and topics they have. This helps to understand high-level grouping and to find meta-clusters in the data.
Conclusion
Topological segmentation of text helps researchers to systematise the knowledge in a vast amount of textual information available right now. While performing the segmentation and analysing each segment, the whole volume of data gets split into clusters, which simplifies the analysis and understanding of the data. The identified keywords and summary sentences help to find the most representative pieces of information and speed up the search for an answer.
Analysis for this article was performed using the DataRefiner platform. We’ve been using this technology in a number of companies not just for text analysis but also for user activity and IoT segmentation. Get in touch if you’d like to learn more or book a demo.
****
DataRefiner.com is a UK based company that specialises in analysis and segmentation of complex data like user activity, sensor or texts. The DataRefiner platform is the result of many years of refining approaches discussed here, but is applied across a wide variety of industries, including aviation, social networks, fraud detection and more. For more information regarding your industry, contact us on ed@datarefiner.com